GIT
Overview
The GIT model was proposed in GIT: A Generative Image-to-text Transformer for Vision and Language by Jianfeng Wang, Zhengyuan Yang, Xiaowei Hu, Linjie Li, Kevin Lin, Zhe Gan, Zicheng Liu, Ce Liu, Lijuan Wang. GIT is a decoder-only Transformer that leverages CLIP’s vision encoder to condition the model on vision inputs besides text. The model obtains state-of-the-art results on image captioning and visual question answering benchmarks.
The abstract from the paper is the following:
In this paper, we design and train a Generative Image-to-text Transformer, GIT, to unify vision-language tasks such as image/video captioning and question answering. While generative models provide a consistent network architecture between pre-training and fine-tuning, existing work typically contains complex structures (uni/multi-modal encoder/decoder) and depends on external modules such as object detectors/taggers and optical character recognition (OCR). In GIT, we simplify the architecture as one image encoder and one text decoder under a single language modeling task. We also scale up the pre-training data and the model size to boost the model performance. Without bells and whistles, our GIT establishes new state of the arts on 12 challenging benchmarks with a large margin. For instance, our model surpasses the human performance for the first time on TextCaps (138.2 vs. 125.5 in CIDEr). Furthermore, we present a new scheme of generation-based image classification and scene text recognition, achieving decent performance on standard benchmarks.
Tips:
- GIT is implemented in a very similar way to GPT-2, the only difference being that the model is also conditioned on
pixel_values
. - One can use GitProcessor to prepare images for the model, and the
generate
method for autoregressive generation.
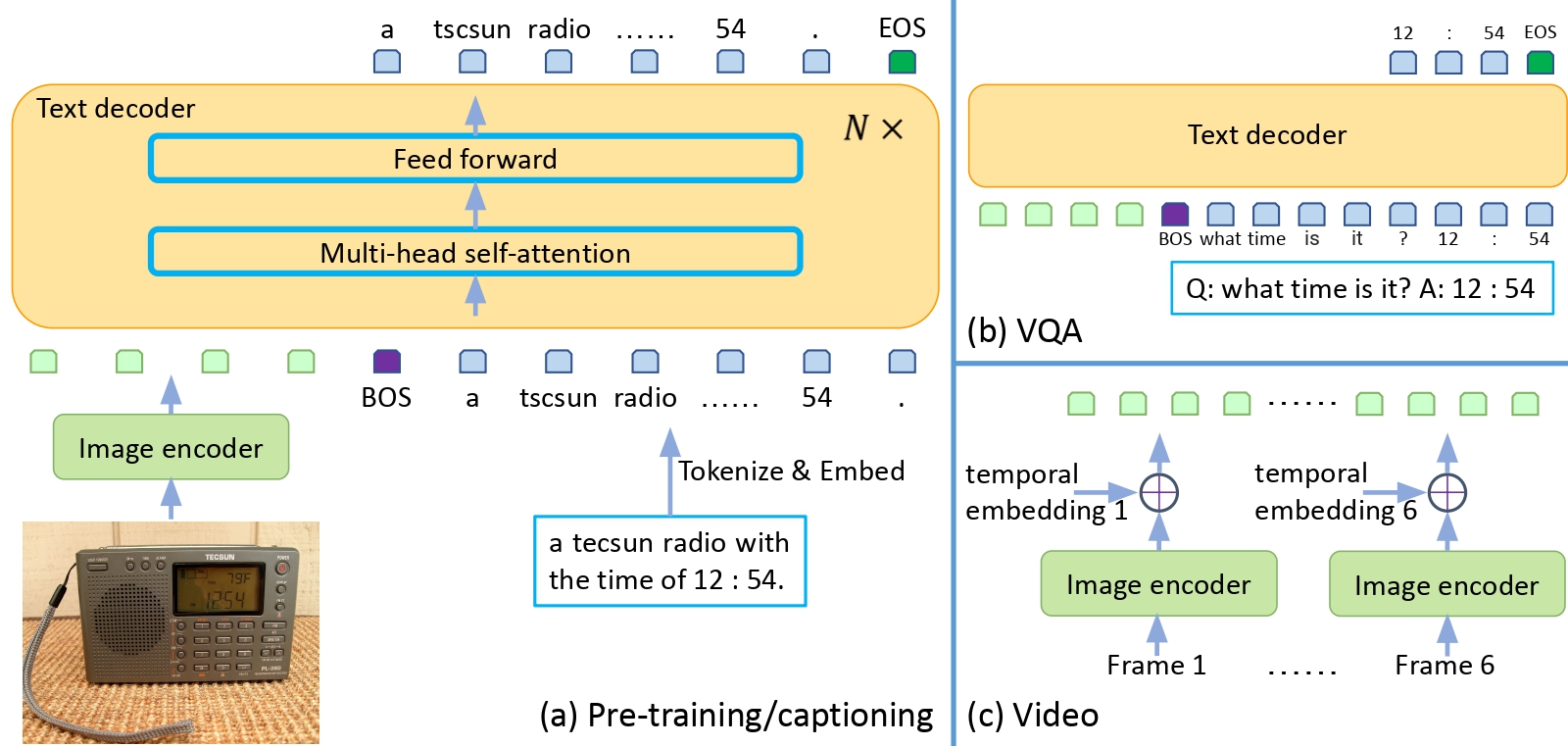
This model was contributed by nielsr. The original code can be found here.
Resources
A list of official Model Database and community (indicated by 🌎) resources to help you get started with GIT.
- Demo notebooks regarding inference + fine-tuning GIT on custom data can be found here.
- See also: Causal language modeling task guide
If you’re interested in submitting a resource to be included here, please feel free to open a Pull Request and we will review it. The resource should ideally demonstrate something new instead of duplicating an existing resource.
GitVisionConfig
class transformers.GitVisionConfig
< source >( hidden_size = 768 intermediate_size = 3072 num_hidden_layers = 12 num_attention_heads = 12 num_channels = 3 image_size = 224 patch_size = 16 hidden_act = 'quick_gelu' layer_norm_eps = 1e-05 attention_dropout = 0.0 initializer_range = 0.02 **kwargs )
Parameters
- hidden_size (
int
, optional, defaults to 768) — Dimensionality of the encoder layers and the pooler layer. -
intermediate_size (
int
, optional, defaults to 3072) — Dimensionality of the “intermediate” (i.e., feed-forward) layer in the Transformer encoder. - num_hidden_layers (
int
, optional, defaults to 12) — Number of hidden layers in the Transformer encoder. -
num_attention_heads (
int
, optional, defaults to 12) — Number of attention heads for each attention layer in the Transformer encoder. -
image_size (
int
, optional, defaults to 224) — The size (resolution) of each image. -
patch_size (
int
, optional, defaults to 16) — The size (resolution) of each patch. - hidden_act (
str
orfunction
, optional, defaults to"quick_gelu"
) — The non-linear activation function (function or string) in the encoder and pooler. If string,"gelu"
,"relu"
,"selu"
and"gelu_new"
`"quick_gelu"
are supported. -
layer_norm_eps (
float
, optional, defaults to 1e-5) — The epsilon used by the layer normalization layers. -
attention_dropout (
float
, optional, defaults to 0.0) — The dropout ratio for the attention probabilities. -
initializer_range (
float
, optional, defaults to 0.02) — The standard deviation of the truncated_normal_initializer for initializing all weight matrices.
This is the configuration class to store the configuration of a GitVisionModel. It is used to instantiate a GIT vision encoder according to the specified arguments, defining the model architecture. Instantiating a configuration with the defaults will yield a similar configuration to that of the vision encoder of the GIT microsoft/git-base architecture.
Configuration objects inherit from PretrainedConfig and can be used to control the model outputs. Read the documentation from PretrainedConfig for more information.
Example:
>>> from transformers import GitVisionConfig, GitVisionModel
>>> # Initializing a GitVisionConfig with microsoft/git-base style configuration
>>> configuration = GitVisionConfig()
>>> # Initializing a GitVisionModel (with random weights) from the microsoft/git-base style configuration
>>> model = GitVisionModel(configuration)
>>> # Accessing the model configuration
>>> configuration = model.config
GitVisionModel
class transformers.GitVisionModel
< source >( config: GitVisionConfig )
Parameters
- config (GitConfig) — Model configuration class with all the parameters of the model. Initializing with a config file does not load the weights associated with the model, only the configuration. Check out the from_pretrained() method to load the model weights.
The vision model from CLIP, used in GIT, without any head or projection on top.
This model inherits from PreTrainedModel. Check the superclass documentation for the generic methods the library implements for all its model (such as downloading or saving, resizing the input embeddings, pruning heads etc.)
This model is also a PyTorch torch.nn.Module subclass. Use it as a regular PyTorch Module and refer to the PyTorch documentation for all matter related to general usage and behavior.
forward
< source >(
pixel_values: typing.Optional[torch.FloatTensor] = None
output_attentions: typing.Optional[bool] = None
output_hidden_states: typing.Optional[bool] = None
return_dict: typing.Optional[bool] = None
)
→
transformers.modeling_outputs.BaseModelOutput or tuple(torch.FloatTensor)
Parameters
-
pixel_values (
torch.FloatTensor
of shape(batch_size, num_channels, height, width)
) — Pixel values. Padding will be ignored by default should you provide it. Pixel values can be obtained using AutoImageProcessor. See CLIPImageProcessor.call() for details. -
output_attentions (
bool
, optional) — Whether or not to return the attentions tensors of all attention layers. Seeattentions
under returned tensors for more detail. - output_hidden_states (
bool
, optional) — Whether or not to return the hidden states of all layers. Seehidden_states
under returned tensors for more detail. -
return_dict (
bool
, optional) — Whether or not to return a ModelOutput instead of a plain tuple.
Returns
transformers.modeling_outputs.BaseModelOutput or tuple(torch.FloatTensor)
A transformers.modeling_outputs.BaseModelOutput or a tuple of
torch.FloatTensor
(if return_dict=False
is passed or when config.return_dict=False
) comprising various
elements depending on the configuration (<class 'transformers.models.git.configuration_git.GitVisionConfig'>
) and inputs.
-
last_hidden_state (
torch.FloatTensor
of shape(batch_size, sequence_length, hidden_size)
) — Sequence of hidden-states at the output of the last layer of the model. -
hidden_states (
tuple(torch.FloatTensor)
, optional, returned whenoutput_hidden_states=True
is passed or whenconfig.output_hidden_states=True
) — Tuple oftorch.FloatTensor
(one for the output of the embeddings, if the model has an embedding layer, + one for the output of each layer) of shape(batch_size, sequence_length, hidden_size)
.Hidden-states of the model at the output of each layer plus the optional initial embedding outputs.
-
attentions (
tuple(torch.FloatTensor)
, optional, returned whenoutput_attentions=True
is passed or whenconfig.output_attentions=True
) — Tuple oftorch.FloatTensor
(one for each layer) of shape(batch_size, num_heads, sequence_length, sequence_length)
.Attentions weights after the attention softmax, used to compute the weighted average in the self-attention heads.
The GitVisionModel forward method, overrides the __call__
special method.
Although the recipe for forward pass needs to be defined within this function, one should call the Module
instance afterwards instead of this since the former takes care of running the pre and post processing steps while
the latter silently ignores them.
Examples:
>>> from PIL import Image
>>> import requests
>>> from transformers import AutoProcessor, GitVisionModel
>>> processor = AutoProcessor.from_pretrained("microsoft/git-base")
>>> model = GitVisionModel.from_pretrained("microsoft/git-base")
>>> url = "http://images.cocodataset.org/val2017/000000039769.jpg"
>>> image = Image.open(requests.get(url, stream=True).raw)
>>> inputs = processor(images=image, return_tensors="pt")
>>> outputs = model(**inputs)
>>> last_hidden_state = outputs.last_hidden_state
GitConfig
class transformers.GitConfig
< source >( vision_config = None vocab_size = 30522 hidden_size = 768 num_hidden_layers = 6 num_attention_heads = 12 intermediate_size = 3072 hidden_act = 'gelu' hidden_dropout_prob = 0.1 attention_probs_dropout_prob = 0.1 max_position_embeddings = 1024 initializer_range = 0.02 layer_norm_eps = 1e-12 pad_token_id = 0 position_embedding_type = 'absolute' use_cache = True tie_word_embeddings = False bos_token_id = 101 eos_token_id = 102 num_image_with_embedding = None **kwargs )
Parameters
-
vision_config (
dict
, optional) — Dictionary of configuration options used to initialize GitVisionConfig. -
vocab_size (
int
, optional, defaults to 30522) — Vocabulary size of the GIT model. Defines the number of different tokens that can be represented by theinputs_ids
passed when calling GitModel. - hidden_size (
int
, optional, defaults to 768) — Dimensionality of the encoder layers and the pooler layer. - num_hidden_layers (
int
, optional, defaults to 6) — Number of hidden layers in the Transformer encoder. -
num_attention_heads (
int
, optional, defaults to 12) — Number of attention heads for each attention layer in the Transformer encoder. -
intermediate_size (
int
, optional, defaults to 3072) — Dimensionality of the “intermediate” (often named feed-forward) layer in the Transformer encoder. - hidden_act (
str
orCallable
, optional, defaults to"gelu"
) — The non-linear activation function (function or string) in the encoder and pooler. If string,"gelu"
,"relu"
,"silu"
and"gelu_new"
are supported. - hidden_dropout_prob (
float
, optional, defaults to 0.1) — The dropout probability for all fully connected layers in the embeddings, encoder, and pooler. -
attention_probs_dropout_prob (
float
, optional, defaults to 0.1) — The dropout ratio for the attention probabilities. -
max_position_embeddings (
int
, optional, defaults to 1024) — The maximum sequence length that this model might ever be used with. Typically set this to something large just in case (e.g., 512 or 1024 or 2048). -
initializer_range (
float
, optional, defaults to 0.02) — The standard deviation of the truncated_normal_initializer for initializing all weight matrices. -
layer_norm_eps (
float
, optional, defaults to 1e-12) — The epsilon used by the layer normalization layers. -
position_embedding_type (
str
, optional, defaults to"absolute"
) — Type of position embedding. Choose one of"absolute"
,"relative_key"
,"relative_key_query"
. For positional embeddings use"absolute"
. For more information on"relative_key"
, please refer to Self-Attention with Relative Position Representations (Shaw et al.). For more information on"relative_key_query"
, please refer to Method 4 in Improve Transformer Models with Better Relative Position Embeddings (Huang et al.). -
use_cache (
bool
, optional, defaults toTrue
) — Whether or not the model should return the last key/values attentions (not used by all models). -
num_image_with_embedding (
int
, optional) — The number of temporal embeddings to add, in case the model is used for video captioning/VQA.
This is the configuration class to store the configuration of a GitModel. It is used to instantiate a GIT model according to the specified arguments, defining the model architecture. Instantiating a configuration with the defaults will yield a similar configuration to that of the GIT microsoft/git-base architecture.
Configuration objects inherit from PretrainedConfig and can be used to control the model outputs. Read the documentation from PretrainedConfig for more information.
Examples:
>>> from transformers import GitConfig, GitModel
>>> # Initializing a GIT microsoft/git-base style configuration
>>> configuration = GitConfig()
>>> # Initializing a model (with random weights) from the microsoft/git-base style configuration
>>> model = GitModel(configuration)
>>> # Accessing the model configuration
>>> configuration = model.config
GitProcessor
class transformers.GitProcessor
< source >( image_processor tokenizer )
Parameters
- image_processor (AutoImageProcessor) — The image processor is a required input.
- tokenizer (AutoTokenizer) — The tokenizer is a required input.
Constructs a GIT processor which wraps a CLIP image processor and a BERT tokenizer into a single processor.
GitProcessor offers all the functionalities of CLIPImageProcessor and BertTokenizerFast. See the
call() and decode()
for more information.
__call__
< source >( text = None images = None return_tensors = None **kwargs ) → BatchEncoding
Parameters
-
text (
str
,List[str]
,List[List[str]]
) — The sequence or batch of sequences to be encoded. Each sequence can be a string or a list of strings (pretokenized string). If the sequences are provided as list of strings (pretokenized), you must setis_split_into_words=True
(to lift the ambiguity with a batch of sequences). -
images (
PIL.Image.Image
,np.ndarray
,torch.Tensor
,List[PIL.Image.Image]
,List[np.ndarray]
,List[torch.Tensor]
) — The image or batch of images to be prepared. Each image can be a PIL image, NumPy array or PyTorch tensor. In case of a NumPy array/PyTorch tensor, each image should be of shape (C, H, W), where C is a number of channels, H and W are image height and width. -
return_tensors (
str
or TensorType, optional) — If set, will return tensors of a particular framework. Acceptable values are:'tf'
: Return TensorFlowtf.constant
objects.'pt'
: Return PyTorchtorch.Tensor
objects.'np'
: Return NumPynp.ndarray
objects.'jax'
: Return JAXjnp.ndarray
objects.
Returns
A BatchEncoding with the following fields:
- input_ids — List of token ids to be fed to a model. Returned when
text
is notNone
. - attention_mask — List of indices specifying which tokens should be attended to by the model (when
return_attention_mask=True
or if “attention_mask” is inself.model_input_names
and iftext
is notNone
). - pixel_values — Pixel values to be fed to a model. Returned when
images
is notNone
.
Main method to prepare for the model one or several sequences(s) and image(s). This method forwards the text
and kwargs
arguments to BertTokenizerFast’s call() if text
is not None
to encode
the text. To prepare the image(s), this method forwards the images
and kwrags
arguments to
CLIPImageProcessor’s call() if images
is not None
. Please refer to the doctsring
of the above two methods for more information.
GitModel
class transformers.GitModel
< source >( config )
Parameters
- config (GitConfig) — Model configuration class with all the parameters of the model. Initializing with a config file does not load the weights associated with the model, only the configuration. Check out the from_pretrained() method to load the model weights.
The bare GIT Model transformer consisting of a CLIP image encoder and text decoder outputting raw hidden-states without any specific head on top.
This model inherits from PreTrainedModel. Check the superclass documentation for the generic methods the library implements for all its model (such as downloading or saving, resizing the input embeddings, pruning heads etc.)
This model is also a PyTorch torch.nn.Module subclass. Use it as a regular PyTorch Module and refer to the PyTorch documentation for all matter related to general usage and behavior.
forward
< source >(
input_ids: typing.Optional[torch.Tensor] = None
attention_mask: typing.Optional[torch.Tensor] = None
position_ids: typing.Optional[torch.Tensor] = None
pixel_values: typing.Optional[torch.Tensor] = None
head_mask: typing.Optional[torch.Tensor] = None
inputs_embeds: typing.Optional[torch.Tensor] = None
past_key_values: typing.Optional[typing.List[torch.FloatTensor]] = None
use_cache: typing.Optional[bool] = None
output_attentions: typing.Optional[bool] = None
output_hidden_states: typing.Optional[bool] = None
return_dict: typing.Optional[bool] = None
)
→
transformers.modeling_outputs.BaseModelOutputWithPooling or tuple(torch.FloatTensor)
Parameters
-
input_ids (
torch.LongTensor
of shape(batch_size, sequence_length)
) — Indices of input sequence tokens in the vocabulary.Indices can be obtained using AutoTokenizer. See PreTrainedTokenizer.encode() and PreTrainedTokenizer.call() for details.
-
attention_mask (
torch.FloatTensor
of shape(batch_size, sequence_length)
, optional) — Mask to avoid performing attention on padding token indices. Mask values selected in[0, 1]
:- 1 for tokens that are not masked,
- 0 for tokens that are masked.
-
position_ids (
torch.LongTensor
of shape(batch_size, sequence_length)
, optional) — Indices of positions of each input sequence tokens in the position embeddings. Selected in the range[0, config.max_position_embeddings - 1]
. -
pixel_values (
torch.FloatTensor
of shape(batch_size, num_channels, height, width)
) — Pixel values. Pixel values can be obtained using AutoImageProcessor. See CLIPImageProcessor.call() for details. -
head_mask (
torch.FloatTensor
of shape(num_heads,)
or(num_layers, num_heads)
, optional) — Mask to nullify selected heads of the self-attention modules. Mask values selected in[0, 1]
:- 1 indicates the head is not masked,
- 0 indicates the head is masked.
-
inputs_embeds (
torch.FloatTensor
of shape(batch_size, sequence_length, hidden_size)
, optional) — Optionally, instead of passinginput_ids
you can choose to directly pass an embedded representation. This is useful if you want more control over how to convertinput_ids
indices into associated vectors than the model’s internal embedding lookup matrix. -
output_attentions (
bool
, optional) — Whether or not to return the attentions tensors of all attention layers. Seeattentions
under returned tensors for more detail. - output_hidden_states (
bool
, optional) — Whether or not to return the hidden states of all layers. Seehidden_states
under returned tensors for more detail. -
return_dict (
bool
, optional) — Whether or not to return a ModelOutput instead of a plain tuple. -
past_key_values (
tuple(tuple(torch.FloatTensor))
of lengthconfig.n_layers
with each tuple having 4 tensors of shape(batch_size, num_heads, sequence_length - 1, embed_size_per_head)
) — Contains precomputed key and value hidden states of the attention blocks. Can be used to speed up decoding.If
past_key_values
are used, the user can optionally input only the lastdecoder_input_ids
(those that don’t have their past key value states given to this model) of shape(batch_size, 1)
instead of alldecoder_input_ids
of shape(batch_size, sequence_length)
. -
use_cache (
bool
, optional) — If set toTrue
,past_key_values
key value states are returned and can be used to speed up decoding (seepast_key_values
).
Returns
transformers.modeling_outputs.BaseModelOutputWithPooling or tuple(torch.FloatTensor)
A transformers.modeling_outputs.BaseModelOutputWithPooling or a tuple of
torch.FloatTensor
(if return_dict=False
is passed or when config.return_dict=False
) comprising various
elements depending on the configuration (GitConfig) and inputs.
-
last_hidden_state (
torch.FloatTensor
of shape(batch_size, sequence_length, hidden_size)
) — Sequence of hidden-states at the output of the last layer of the model. -
pooler_output (
torch.FloatTensor
of shape(batch_size, hidden_size)
) — Last layer hidden-state of the first token of the sequence (classification token) after further processing through the layers used for the auxiliary pretraining task. E.g. for BERT-family of models, this returns the classification token after processing through a linear layer and a tanh activation function. The linear layer weights are trained from the next sentence prediction (classification) objective during pretraining. -
hidden_states (
tuple(torch.FloatTensor)
, optional, returned whenoutput_hidden_states=True
is passed or whenconfig.output_hidden_states=True
) — Tuple oftorch.FloatTensor
(one for the output of the embeddings, if the model has an embedding layer, + one for the output of each layer) of shape(batch_size, sequence_length, hidden_size)
.Hidden-states of the model at the output of each layer plus the optional initial embedding outputs.
-
attentions (
tuple(torch.FloatTensor)
, optional, returned whenoutput_attentions=True
is passed or whenconfig.output_attentions=True
) — Tuple oftorch.FloatTensor
(one for each layer) of shape(batch_size, num_heads, sequence_length, sequence_length)
.Attentions weights after the attention softmax, used to compute the weighted average in the self-attention heads.
The GitModel forward method, overrides the __call__
special method.
Although the recipe for forward pass needs to be defined within this function, one should call the Module
instance afterwards instead of this since the former takes care of running the pre and post processing steps while
the latter silently ignores them.
Examples:
>>> from transformers import AutoProcessor, AutoModel
>>> import requests
>>> from PIL import Image
>>> processor = AutoProcessor.from_pretrained("microsoft/git-base")
>>> model = AutoModel.from_pretrained("microsoft/git-base")
>>> url = "http://images.cocodataset.org/val2017/000000039769.jpg"
>>> image = Image.open(requests.get(url, stream=True).raw)
>>> text = "this is an image of two cats"
>>> inputs = processor(text, images=image, return_tensors="pt")
>>> outputs = model(**inputs)
>>> last_hidden_state = outputs.last_hidden_state
GitForCausalLM
class transformers.GitForCausalLM
< source >( config )
Parameters
- config (GitConfig) — Model configuration class with all the parameters of the model. Initializing with a config file does not load the weights associated with the model, only the configuration. Check out the from_pretrained() method to load the model weights.
GIT Model with a language modeling
head on top for autoregressive language modeling.
This model inherits from PreTrainedModel. Check the superclass documentation for the generic methods the library implements for all its model (such as downloading or saving, resizing the input embeddings, pruning heads etc.)
This model is also a PyTorch torch.nn.Module subclass. Use it as a regular PyTorch Module and refer to the PyTorch documentation for all matter related to general usage and behavior.
forward
< source >(
input_ids: typing.Optional[torch.Tensor] = None
attention_mask: typing.Optional[torch.Tensor] = None
position_ids: typing.Optional[torch.Tensor] = None
pixel_values: typing.Optional[torch.Tensor] = None
head_mask: typing.Optional[torch.Tensor] = None
inputs_embeds: typing.Optional[torch.Tensor] = None
labels: typing.Optional[torch.Tensor] = None
past_key_values: typing.Optional[typing.List[torch.Tensor]] = None
use_cache: typing.Optional[bool] = None
output_attentions: typing.Optional[bool] = None
output_hidden_states: typing.Optional[bool] = None
return_dict: typing.Optional[bool] = None
)
→
transformers.modeling_outputs.CausalLMOutputWithPast or tuple(torch.FloatTensor)
Parameters
-
input_ids (
torch.LongTensor
of shape(batch_size, sequence_length)
) — Indices of input sequence tokens in the vocabulary.Indices can be obtained using AutoTokenizer. See PreTrainedTokenizer.encode() and PreTrainedTokenizer.call() for details.
-
attention_mask (
torch.FloatTensor
of shape(batch_size, sequence_length)
, optional) — Mask to avoid performing attention on padding token indices. Mask values selected in[0, 1]
:- 1 for tokens that are not masked,
- 0 for tokens that are masked.
-
position_ids (
torch.LongTensor
of shape(batch_size, sequence_length)
, optional) — Indices of positions of each input sequence tokens in the position embeddings. Selected in the range[0, config.max_position_embeddings - 1]
. -
pixel_values (
torch.FloatTensor
of shape(batch_size, num_channels, height, width)
) — Pixel values. Pixel values can be obtained using AutoImageProcessor. See CLIPImageProcessor.call() for details. -
head_mask (
torch.FloatTensor
of shape(num_heads,)
or(num_layers, num_heads)
, optional) — Mask to nullify selected heads of the self-attention modules. Mask values selected in[0, 1]
:- 1 indicates the head is not masked,
- 0 indicates the head is masked.
-
inputs_embeds (
torch.FloatTensor
of shape(batch_size, sequence_length, hidden_size)
, optional) — Optionally, instead of passinginput_ids
you can choose to directly pass an embedded representation. This is useful if you want more control over how to convertinput_ids
indices into associated vectors than the model’s internal embedding lookup matrix. -
output_attentions (
bool
, optional) — Whether or not to return the attentions tensors of all attention layers. Seeattentions
under returned tensors for more detail. - output_hidden_states (
bool
, optional) — Whether or not to return the hidden states of all layers. Seehidden_states
under returned tensors for more detail. -
return_dict (
bool
, optional) — Whether or not to return a ModelOutput instead of a plain tuple. -
labels (
torch.LongTensor
of shape(batch_size, sequence_length)
, optional) — Labels for computing the left-to-right language modeling loss (next word prediction). Indices should be in[-100, 0, ..., config.vocab_size]
(seeinput_ids
docstring) Tokens with indices set to-100
are ignored (masked), the loss is only computed for the tokens with labels n[0, ..., config.vocab_size]
-
past_key_values (
tuple(tuple(torch.FloatTensor))
of lengthconfig.n_layers
with each tuple having 4 tensors of shape(batch_size, num_heads, sequence_length - 1, embed_size_per_head)
) — Contains precomputed key and value hidden states of the attention blocks. Can be used to speed up decoding.If
past_key_values
are used, the user can optionally input only the lastdecoder_input_ids
(those that don’t have their past key value states given to this model) of shape(batch_size, 1)
instead of alldecoder_input_ids
of shape(batch_size, sequence_length)
. -
use_cache (
bool
, optional) — If set toTrue
,past_key_values
key value states are returned and can be used to speed up decoding (seepast_key_values
).
Returns
transformers.modeling_outputs.CausalLMOutputWithPast or tuple(torch.FloatTensor)
A transformers.modeling_outputs.CausalLMOutputWithPast or a tuple of
torch.FloatTensor
(if return_dict=False
is passed or when config.return_dict=False
) comprising various
elements depending on the configuration (GitConfig) and inputs.
-
loss (
torch.FloatTensor
of shape(1,)
, optional, returned whenlabels
is provided) — Language modeling loss (for next-token prediction). -
logits (
torch.FloatTensor
of shape(batch_size, sequence_length, config.vocab_size)
) — Prediction scores of the language modeling head (scores for each vocabulary token before SoftMax). -
past_key_values (
tuple(tuple(torch.FloatTensor))
, optional, returned whenuse_cache=True
is passed or whenconfig.use_cache=True
) — Tuple oftuple(torch.FloatTensor)
of lengthconfig.n_layers
, with each tuple having 2 tensors of shape(batch_size, num_heads, sequence_length, embed_size_per_head)
)Contains pre-computed hidden-states (key and values in the self-attention blocks) that can be used (see
past_key_values
input) to speed up sequential decoding. -
hidden_states (
tuple(torch.FloatTensor)
, optional, returned whenoutput_hidden_states=True
is passed or whenconfig.output_hidden_states=True
) — Tuple oftorch.FloatTensor
(one for the output of the embeddings, if the model has an embedding layer, + one for the output of each layer) of shape(batch_size, sequence_length, hidden_size)
.Hidden-states of the model at the output of each layer plus the optional initial embedding outputs.
-
attentions (
tuple(torch.FloatTensor)
, optional, returned whenoutput_attentions=True
is passed or whenconfig.output_attentions=True
) — Tuple oftorch.FloatTensor
(one for each layer) of shape(batch_size, num_heads, sequence_length, sequence_length)
.Attentions weights after the attention softmax, used to compute the weighted average in the self-attention heads.
The GitForCausalLM forward method, overrides the __call__
special method.
Although the recipe for forward pass needs to be defined within this function, one should call the Module
instance afterwards instead of this since the former takes care of running the pre and post processing steps while
the latter silently ignores them.
Examples:
Image captioning example:
>>> from transformers import AutoProcessor, AutoModelForCausalLM
>>> import requests
>>> from PIL import Image
>>> processor = AutoProcessor.from_pretrained("microsoft/git-base-coco")
>>> model = AutoModelForCausalLM.from_pretrained("microsoft/git-base-coco")
>>> url = "http://images.cocodataset.org/val2017/000000039769.jpg"
>>> image = Image.open(requests.get(url, stream=True).raw)
>>> pixel_values = processor(images=image, return_tensors="pt").pixel_values
>>> generated_ids = model.generate(pixel_values=pixel_values, max_length=50)
>>> generated_caption = processor.batch_decode(generated_ids, skip_special_tokens=True)[0]
>>> print(generated_caption)
two cats sleeping on a pink blanket next to remotes.
Visual question answering (VQA) example:
>>> from transformers import AutoProcessor, AutoModelForCausalLM
>>> from huggingface_hub import hf_hub_download
>>> from PIL import Image
>>> processor = AutoProcessor.from_pretrained("microsoft/git-base-textvqa")
>>> model = AutoModelForCausalLM.from_pretrained("microsoft/git-base-textvqa")
>>> file_path = hf_hub_download(repo_id="nielsr/textvqa-sample", filename="bus.png", repo_type="dataset")
>>> image = Image.open(file_path).convert("RGB")
>>> pixel_values = processor(images=image, return_tensors="pt").pixel_values
>>> question = "what does the front of the bus say at the top?"
>>> input_ids = processor(text=question, add_special_tokens=False).input_ids
>>> input_ids = [processor.tokenizer.cls_token_id] + input_ids
>>> input_ids = torch.tensor(input_ids).unsqueeze(0)
>>> generated_ids = model.generate(pixel_values=pixel_values, input_ids=input_ids, max_length=50)
>>> print(processor.batch_decode(generated_ids, skip_special_tokens=True))
['what does the front of the bus say at the top? special']
Video captioning example:
>>> import av
>>> import numpy as np
>>> from PIL import Image
>>> from huggingface_hub import hf_hub_download
>>> from transformers import AutoProcessor, AutoModelForCausalLM
>>> processor = AutoProcessor.from_pretrained("microsoft/git-base-vatex")
>>> model = AutoModelForCausalLM.from_pretrained("microsoft/git-base-vatex")
>>> # set seed for reproducability
>>> np.random.seed(45)
>>> def read_video_pyav(container, indices):
... '''
... Decode the video with PyAV decoder.
... Args:
... container (`av.container.input.InputContainer`): PyAV container.
... indices (`List[int]`): List of frame indices to decode.
... Returns:
... result (np.ndarray): np array of decoded frames of shape (num_frames, height, width, 3).
... '''
... frames = []
... container.seek(0)
... start_index = indices[0]
... end_index = indices[-1]
... for i, frame in enumerate(container.decode(video=0)):
... if i > end_index:
... break
... if i >= start_index and i in indices:
... frames.append(frame)
... return np.stack([x.to_ndarray(format="rgb24") for x in frames])
>>> def sample_frame_indices(clip_len, frame_sample_rate, seg_len):
... '''
... Sample a given number of frame indices from the video.
... Args:
... clip_len (`int`): Total number of frames to sample.
... frame_sample_rate (`int`): Sample every n-th frame.
... seg_len (`int`): Maximum allowed index of sample's last frame.
... Returns:
... indices (`List[int]`): List of sampled frame indices
... '''
... converted_len = int(clip_len * frame_sample_rate)
... end_idx = np.random.randint(converted_len, seg_len)
... start_idx = end_idx - converted_len
... indices = np.linspace(start_idx, end_idx, num=clip_len)
... indices = np.clip(indices, start_idx, end_idx - 1).astype(np.int64)
... return indices
>>> # load video
>>> file_path = hf_hub_download(
... repo_id="nielsr/video-demo", filename="eating_spaghetti.mp4", repo_type="dataset"
... )
>>> container = av.open(file_path)
>>> # sample frames
>>> num_frames = model.config.num_image_with_embedding
>>> indices = sample_frame_indices(
... clip_len=num_frames, frame_sample_rate=4, seg_len=container.streams.video[0].frames
... )
>>> frames = read_video_pyav(container, indices)
>>> pixel_values = processor(images=list(frames), return_tensors="pt").pixel_values
>>> generated_ids = model.generate(pixel_values=pixel_values, max_length=50)
>>> print("Generated caption:", processor.batch_decode(generated_ids, skip_special_tokens=True))
Generated caption: ['a woman is sitting at a table and she is talking about the food she is holding.']